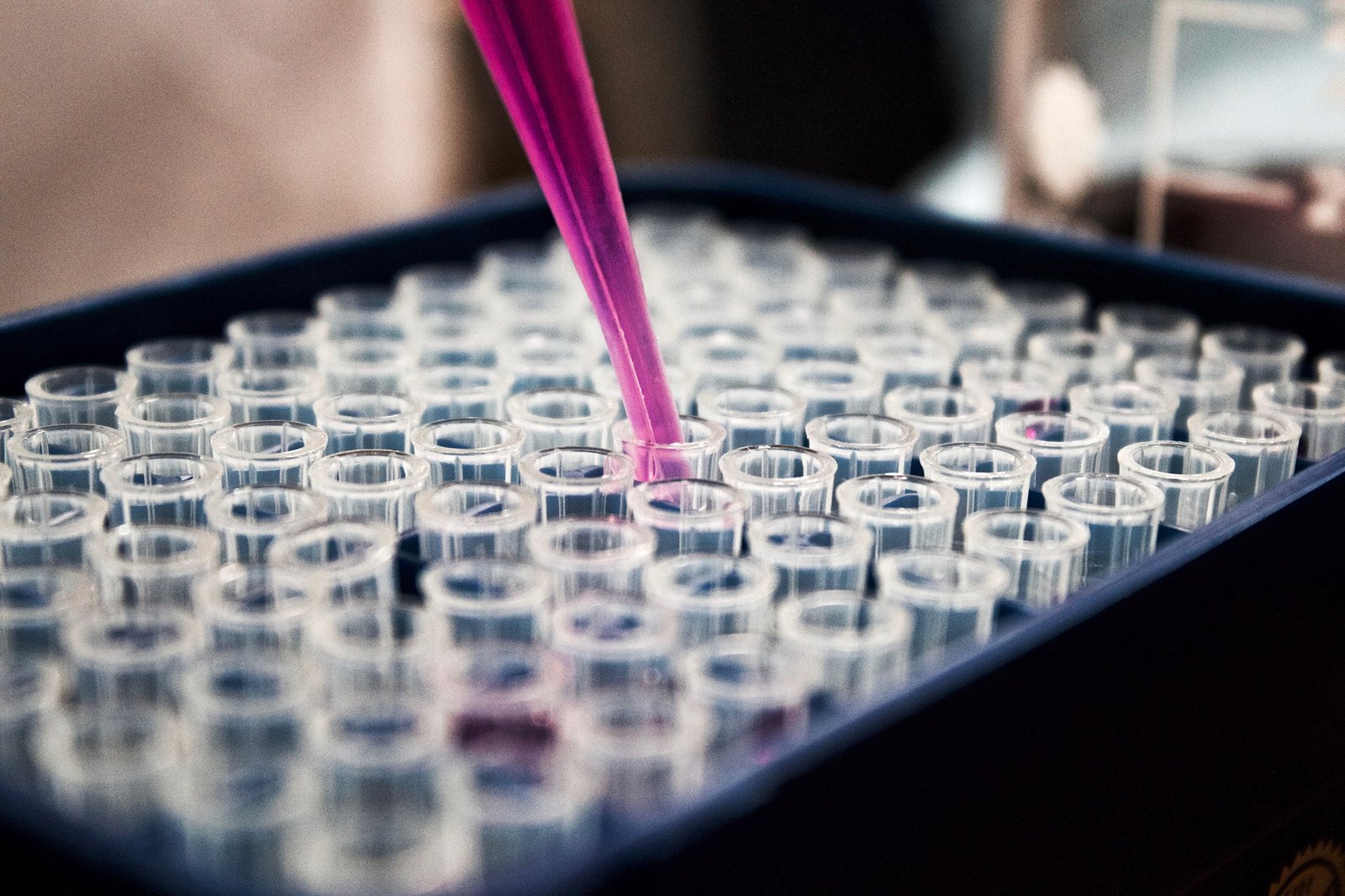
AI-Powered Healthcare Diagnostics: Transformative Tools for Disease Diagnosis
In the realm of healthcare, the integration of technology has led to groundbreaking advancements, particularly in the field of disease diagnosis. Diagnostic Assistance Tools, driven by artificial intelligence (AI) and machine learning algorithms, have emerged as powerful allies for healthcare professionals. These tools enhance the accuracy, speed, and efficiency of disease diagnosis, revolutionizing patient care. In this exploration, we'll delve into the world of Diagnostic Assistance Tools, providing multiple examples to illustrate their impact and paving the way for a solution-oriented approach to the future of healthcare diagnostics.
The Imperative for Advanced Diagnostic Tools
Accurate and timely diagnosis is a cornerstone of effective healthcare. However, the complexity of medical data, the sheer volume of information, and the need for swift decision-making present challenges for healthcare professionals. Diagnostic Assistance Tools leverage AI to analyze vast datasets, detect patterns, and provide valuable insights, offering a comprehensive approach to disease diagnosis.
Examples of Diagnostic Assistance Tools
IBM Watson for Oncology:
IBM Watson for Oncology is an AI-powered platform designed to assist oncologists in cancer treatment decisions. It analyzes medical literature, clinical trial data, and patient records to provide evidence-based recommendations for personalized cancer care.
Google's DeepMind in Ophthalmology:
DeepMind, a subsidiary of Google’s parent company Alphabet, has developed AI algorithms for diagnosing eye diseases. In collaboration with Moorfields Eye Hospital in London, DeepMind’s technology analyzes retinal scans to detect conditions such as macular degeneration and diabetic retinopathy.
PathAI for Pathology:
- PathAI utilizes AI in pathology to aid pathologists in diagnosing diseases from medical images. The platform assists in the identification of patterns and anomalies in pathology slides, leading to more accurate and efficient diagnoses.
IDx-DR for Diabetic Retinopathy:
- IDx-DR is an FDA-approved autonomous AI system that detects diabetic retinopathy in retinal images. The system can provide diagnostic results without the need for a human interpreter, enabling timely intervention for patients with diabetes.
How Diagnostic Assistance Tools Enhance Disease Diagnosis
1. Data Analysis at Scale:
- Diagnostic Assistance Tools can process and analyze vast amounts of medical data, including patient records, medical images, and clinical research. This capability allows for a more comprehensive evaluation of a patient’s health status.
2. Pattern Recognition and Prediction:
- AI algorithms excel in pattern recognition, enabling Diagnostic Assistance Tools to identify subtle indicators of diseases that might be challenging for human practitioners to detect. These tools can also predict disease progression based on historical data.
3. Personalized Medicine:
- By analyzing patient data, including genetic information, Diagnostic Assistance Tools contribute to the realization of personalized medicine. Tailored treatment plans and interventions based on individual characteristics improve the efficacy of medical care.
4. Time-Efficient Diagnoses:
- The speed at which AI algorithms process information significantly reduces the time required for disease diagnosis. Rapid results enable healthcare professionals to initiate timely treatments and interventions, particularly in critical situations.
Real-world Examples of Diagnostic Assistance Tools
1. Holmusk's MindLinc for Mental Health:
- Holmusk’s MindLinc leverages machine learning to assist healthcare providers in diagnosing and treating mental health conditions. By analyzing patient data, including electronic health records and behavioral data, the tool aids in the early detection and management of mental health disorders.
2. Tempus for Cancer Care:
- Tempus uses AI to analyze clinical and molecular data to support oncologists in cancer care decisions. The platform assists in identifying potential clinical trial opportunities, offering insights into optimal treatment strategies for individual patients.
3. Butterfly iQ+ for Ultrasound Imaging:
- The Butterfly iQ+ is an AI-powered handheld ultrasound device that assists healthcare professionals in obtaining high-quality imaging for various medical applications. The device incorporates AI algorithms to enhance image quality and support diagnostic decision-making.
Challenges and Future Prospects
While Diagnostic Assistance Tools showcase remarkable potential, challenges such as ensuring data privacy, addressing algorithm biases, and integrating these tools seamlessly into healthcare workflows need careful consideration. The ongoing evolution of technology and the need for regulatory frameworks also play a crucial role in shaping the future landscape of diagnostic assistance in healthcare.
The Path Forward: Crafting Innovative Solutions
Comprehensive Diagnostic Assistance Solutions
Regulatory Standards and Oversight:
- The establishment of robust regulatory standards and oversight is essential to ensure the safety, accuracy, and ethical use of Diagnostic Assistance Tools. Regulatory frameworks should address data privacy, algorithm transparency, and overall tool reliability.
Interdisciplinary Collaboration:
- Collaboration between healthcare professionals, data scientists, and technology experts is crucial for the development and refinement of Diagnostic Assistance Tools. Interdisciplinary teams can ensure that these tools align with clinical needs and ethical considerations.
Continual Training and Validation:
- Diagnostic Assistance Tools should undergo continual training and validation to ensure their accuracy and reliability across diverse patient populations. Regular updates based on real-world data contribute to the ongoing refinement of these tools.
Patient-Centric Design:
- The design and implementation of Diagnostic Assistance Tools should prioritize the patient experience. This involves transparent communication with patients about the use of AI in their healthcare, ensuring informed consent, and addressing concerns related to data security and privacy.
Conclusion
Diagnostic Assistance Tools represent a paradigm shift in healthcare, offering the potential to revolutionize disease diagnosis. Real-world examples from IBM Watson for Oncology, Google’s DeepMind, PathAI, and IDx-DR demonstrate the diverse applications of AI in enhancing diagnostic capabilities. As we navigate the future of healthcare, challenges and opportunities coexist.
The path forward involves crafting innovative solutions, including regulatory standards and oversight, interdisciplinary collaboration, continual training and validation, and a patient-centric design approach. The question remains: What groundbreaking solutions will emerge as Diagnostic Assistance Tools continue to evolve, and how will they redefine the landscape of disease diagnosis?
For those eager to explore the future of diagnostic assistance in healthcare and discover innovative Artificial Intelligence Development solutions, to implement.